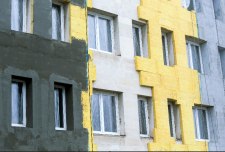
In any industrial process, data processing is becoming more and more important for decision making. Whether it is carried out by complex artificial intelligence systems or more traditional analysis methods, to make these decisions reliable, the data needs to be qualified and the estimates obtained accompanied by confidence indicators.
How can we be sure that the uncertainty linked to the measurements made will allow us to make the right decisions? Will this Design of Experiments, this AI or this Deep Learning algorithm meet the need? LNE can help you answer these questions thanks to its wide-ranging expertise in data science, from the assessment of measurement uncertainty to the evaluation of artificial intelligence systems, including the implementation of experimental designs, statistical analyses and the provision of software and online applications.
Measuring, testing, calibrating, companies and laboratories produce a lot of data every day. To make them usable and intelligible, the quality of their processing is a key step in their development. For decision making, data and measurements also need to be qualified and accompanied by confidence indicators such as measurement uncertainty.
The evaluation of the uncertainty of measurement is the quantification of the doubt associated with a measurement, an indicator of its quality which reflects the knowledge that the user has of a quantity after a measurement. This confidence index will allow him to make decisions by appreciating the associated risks.
Measurement is LNE's core business. As an expert, it helps to draw up international standards in this field and develops its expertise through research programmes. Within the Bureau International des Poids et Mesures (BIPM), it contributes to the development of the International Vocabulary of Metrology (VIM) and the Guide to the Expression of Uncertainty in Measurement (GUM).
Our support is available through :
Accelerating the energy renovation of buildings is a major challenge for reducing greenhouse gas emissions. LNE is working with major national players in the sector (CSTB, CEREMA, Gustave Eiffel University, etc.) to improve the assessment and verification of the energy performance of both new high-performance buildings and existing buildings, before and after renovation. It also contributes to the methodological work carried out within the Fondation Bâtiment Energie (FBE).
More specifically, LNE has contributed to the development of a method for measuring the in-situ thermal resistance of building walls (ANR RESBATI) and to the evaluation of the associated uncertainty. By choosing an active method based on the thermal excitation of the wall, the measurement of thermal resistance is carried out indirectly from the observation of temperature and flow measurements, and requires the use of a thermal model within the framework of a Bayesian inversion procedure.
Every day, conformity decisions are made on the basis of measurement results, to declare the conformity of a measuring instrument to a Maximum Tolerated Error (MTE) following a calibration or to certify the conformity of a product to a regulatory specification. These decisions are inevitably accompanied by a risk, a decision error due to the measurement uncertainty.
To calculate these risks and make decisions with confidence, CaSoft software has been developed as part of a European project (EMPIR 17SIP05 CASoft) led by LNE. The CaSoft software is available for free download on our website.
The implementation of experimental designs is an efficient method to organize and/or optimize tests in the industry. They can be used in particular to evaluate the sources of uncertainty related to a particular measurement process or numerical simulations.
Design of experiments can be used to assist in the evaluation of measurement uncertainties. Coupled with a statistical analysis of the results, they allow to determine the factors to be taken into account in the evaluation of the uncertainty. In numerical simulation, they allow to reduce development costs.
Issues | Solutions | ||
---|---|---|---|
Evaluate an operator effect, detect the effect of an influential factor or find an optimum setting to minimize uncertainty. | ![]()
|
Design of experiments specific to the customer's needs coupled with a statistical analysis of the results obtained. The analysis of variance allows to identify, for example, if the operator effect is significant and must be taken into account in the evaluation of the uncertainty. | |
Evaluate the performance of numerical codes in the field of fire simulation | ![]() |
Implementation of numerical experimental designs to evaluate the probability of exceeding regulatory thresholds associated with critical fire parameters. |
The industrial world is increasingly using machine learning or statistical learning, also known as Deep Learning, to process large volumes of data or complex tasks. To meet the needs of industry, LNE implements state-of-the-art Deep Learning algorithms.
But one of the major problems is the confidence in the predictions provided by these algorithms. This is why the research teams are also working on the development of an innovative methodology for evaluating the uncertainty associated with the predictions of these algorithms from the Deep Learning community.
"The technological breakthrough associated with nanoscience and nanotechnology lies in the tools to be developed to image, analyze and measure matter at this reduced scale" [LNE-NanoTech]. In this context, LNE's research teams are implementing deep learning algorithms to characterize nanomaterial populations on scanning electron microscopy samples: segmentation, classification and completion of particles on each sample. These new methods not only drastically reduce the processing time per sample, but above all open the way to new developments aimed at more precisely characterizing the populations of particles present in consumer products: food, dyes, paints, etc.
Find all our software in our "Software" section
LNE-Matics: software suite designed for data mining and system evaluation
LNE-MCM: evaluate measurement uncertainty by propagation of distributions using Monte Carlo simulations
LNE-RegPoly: integrate the uncertainties associated with standard values and indications to evaluate measurement results and uncertainty
CaSoft: manage the risks associated with conformity assessment when measurement uncertainty must be taken into account
The use of artificial intelligence (AI) systems is accelerating in civil society and industry, promising more effective decision-making in increasingly complex environments. While these systems are generally offered as off-the-shelf services, their suitability for the needs of their users can be questionable.
To help both the public and private sectors to choose an appropriate AI solution, LNE has been building up unique expertise in the evaluation of Artificial Intelligence systems for over 10 years. Thanks to its numerous R&D projects on the subject, it has developed and disseminated tools, methods and standards, in particular through participation in national, European and international AI standardization committees.
LNE can provide solutions to any question related to the choice or performance of an Artificial Intelligence system.
The performance of an AI system is determined by its ability to produce the expected results when faced with unknown data but included in the nominal operating domain of the system. It is therefore necessary to characterize this operating domain and to evaluate its adequacy with the customer's specifications. An AI solution can thus be evaluated on a dataset provided by the customer, which LNE will use to perform a detailed analysis of the system's behavior using the state-of-the-art metrics best suited to the type of data and the task in question.
The robustness analysis enriches the performance analysis with an evaluation of the system's ability to operate in difficult environmental conditions. Robustness focuses on measuring the ability of the system to maintain a satisfactory level of performance when faced with inputs at the limits of its operating domain. These data can be provided by the customer or produced by LNE, in particular by simulating meteorological effects (in the case of images) or sound effects (for systems based on audio inputs).
A third aspect of the evaluation of an AI system consists in characterizing its resilience, in particular when it may have to operate in a degraded mode, i.e. when it encounters inputs outside its nominal operating domain during its life cycle. This can happen when certain technological components of the AI system's information processing chain fail. LNE is able to produce this type of alteration if the customer does not provide its own data.
These three types of analysis can be performed individually or in groups, depending on the needs expressed.
The performance analysis of an AI system designed for people detection in scenes consists in submitting an arbitrary number of clear scenes (well visible people or on the contrary empty sceneries, sufficient luminosity, etc.) to the system and in analyzing the errors committed by this one (a person detected by error or on the contrary not detected). The robustness analysis consists in presenting the system with scenes in low luminosity or taken in bad environmental conditions (night, rain or fog). The resilience analysis is measured by submitting to the system images with dead pixels or blur, corresponding to an optical sensor defect.
As artificial intelligence becomes more and more popular, it becomes common for end-users of intelligent technologies to find themselves facing several competing commercial solutions that are apparently capable of meeting their needs. In this case, the comparison of the performance of each solution according to a common reference (of data and metrics) built from the customer's need allows to identify the most adapted solution in a reliable way.
When the customer's needs are specific, it may be difficult to identify pre-existing solutions, and it may even be necessary to request the creation of a custom solution. Organizing an evaluation campaign then allows to obtain a panorama of the best experts of the task at hand and to obtain a clear vision of the state of the art corresponding to the customer's needs.
In some critical systems simply receiving the decision from an AI system is not enough. It must come with an explanation describing the elements taken into account by the system to reach its conclusion. Very few systems natively integrate explainability functionalities and it is therefore often necessary to resort to a battery of external tools to obtain these answers, which raises the question of their reliability.
LNE positions itself as an evaluator of these external tools, in order to assure its clients of the relevance of the explanations returned. In addition, LNE develops its own in-house explicability tools to give additional depth to its evaluations and to meet the needs of customers who wish to have their AI explained by a trusted third party.
All our detailed services for the evaluation of artificial intelligence systems
In order to meet the current and future challenges of AI evaluation, LNE has initiated the construction of the LEIA platform (Laboratory for the Evaluation of Artificial Intelligence). This is a unique device in the world because of iIts genericity makes it a one-of-a-kind platform in the world. It is designed to evaluate any robot with embedding AI modules (for a global evaluation), such as civil and military intervention robots, personal assistance robots, and infrastructure inspection and maintenance robots. It also allows the evaluation of isolated AI modules (for a more precise evaluation of a single robot component for example).
>> Learn more about the LEIA platform (in French)
To go further on the evaluation of Artificial Intelligences, see our Evaluating Artificial Intelligences folder.
Do you have data processing needs?